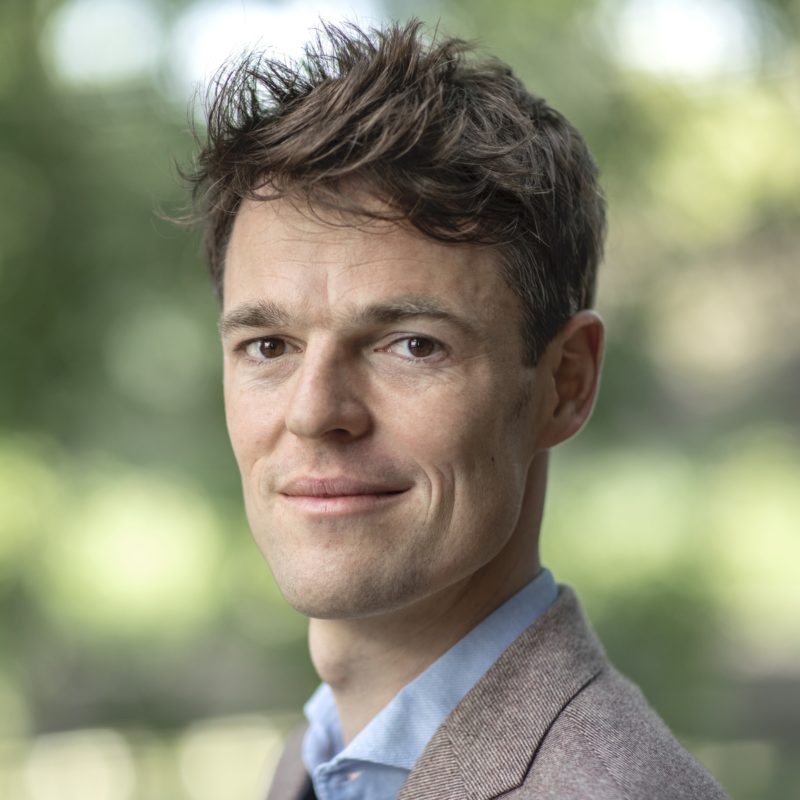
Speakers:
Improving Mental Health Treatment by Balancing Privacy, Interpretability and Predictive Power: a Distributed K-Nearest Neighbors Example
Date:
Tuesday, May 12, 2020
Time:
11:00 am
Summary:
Besides having good predictive power, data science applications in healthcare need to satisfy strict privacy regulations, and preferably be interpretable to both the healthcare professional and, ideally, the patient. As satisfying each of these criteria simultaneously is not straightforward, successful data science applications in healthcare generally require going beyond the standard machine learning approach. This case study provides a practical example of how to augment the standard machine learning approach in order to satisfy the different criteria relevant to clinical practice.
Five mental healthcare institutions in the Netherlands developed a tool to predict treatment outcomes for anxiety and depression, supporting healthcare professionals and patients in deciding whether to continue or adjust treatment. Distributed learning was applied to help satisfy GDPR regulations while preserving as much detail as possible in the available data. K-Nearest Neighbors (KNN) was chosen as a simple, intuitive, visualizable prediction algorithm to promote interpretability among healthcare professionals and patients. KNN was adjusted in order to increase the level of sophistication and therefore the predictive power of the algorithm. Different measures were taken to ensure that the possibility to recognize individual patients was minimized for this specific approach.